Statistics Collaborative is part of WCGclinical.com
If you were trying to reach statcollab.com, you’re in the right place!
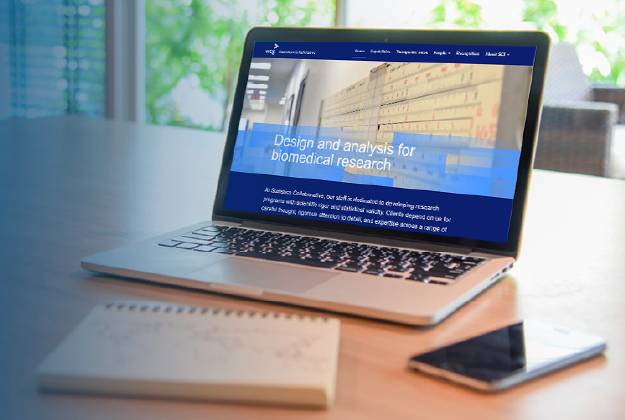
Optimize your Clinical Trial Operations with WCG’s Integrated Solutions
As part of our ongoing efforts to simplify and improve our client experience, we have consolidated the Statistics Collaborative website to wcgclinical.com.
This does not represent any changes to our service to you, and there are no actions you or your teams need to take at this time.
Below, you’ll find links to our solutions, and important resources.
Thank you for the opportunity to support you in your important mission. If you have any questions or concerns, please contact us.
Explore WCG Clinical Endpoint Solutions
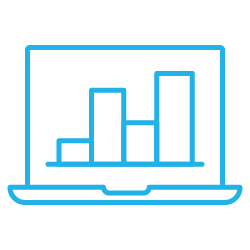
Statistical Consulting
Our statistical consulting services enrich clinical development plans for sponsors who need additional statistical capabilities. This includes protocol and trial design, statistical analysis plan development, and statistical oversight and guidance.
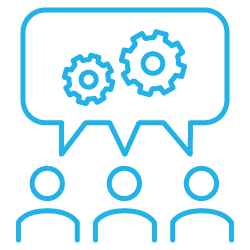
Trial Design & Protocol Planning
Our clinical, medical, and statistical experts improve the quality of your study design, ensure you are identifying the right patient populations and endpoints, reduce the frequency of protocol amendments, inform your decisions, and increase the overall validity and quality of your studies.
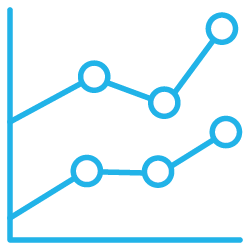
Data Monitoring Committee
Our managed Data Monitoring Committees deliberate over un-blinded data to provide recommendations on how trials should proceed. Our streamlined approach ensures patient safety oversight and effective decision-making.
Important Resources
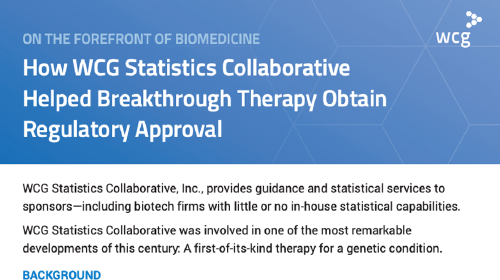
How WCG Statistics Collaborative Helped Breakthrough Therapy Obtain Regulatory Approval
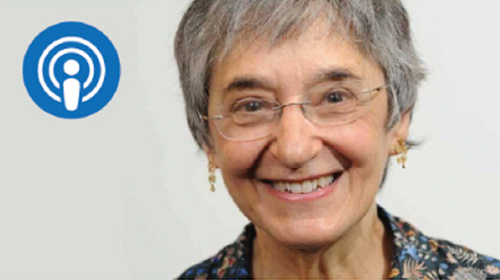
Biostatistics and Biomarkers for the Reliability and Efficiency of Clinical Trials: A Conversation with Dr. Janet Wittes
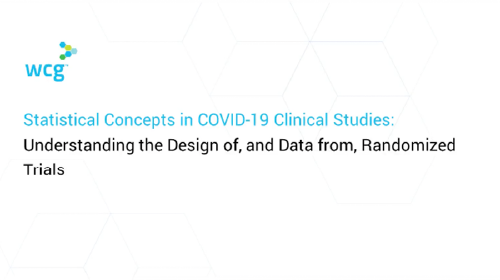
Statistical Concepts in COVID-19 Clinical Studies: Understanding the Design of, and Data from, Randomized Trials
Contact Us
Have a question or want to speak with our sales or support teams?
Use this form to contact us.
Office Information:
212 Carnegie Center, Suite 301
Princeton, NJ 08540
Office: 609.945.0101